【3】数据分析-4-1-由煮面条到图论到图论--NetworkX
今晚跟室友煮了个面条,满满的一锅面条,煮了好久,可是那些缠在一块的面条中间的那部分怎么都煮不熟,着实令人着急。一边煮面条,我就在想这一锅面条不正是一大堆引物放在一个管子里面做Multiplex PCR吗?有的引物可以放在一起煮,有的不能放在一起。那怎样可以满足尽可能减少试管,同时又能满足均一化呢?
一、NetworkX概论
NetworkX是一个用Python语言开发的图论与复杂网络建模工具,内置了常用的图与复杂网络分析算法,可以方便的进行复杂网络数据分析、仿真建模等工作。networkx支持创建简单无向图、有向图和多重图(multigraph);内置许多标准的图论算法,节点可为任意数据;支持任意的边值维度,功能丰富,简单易用。
官网:http://networkx.github.io/index.html
官网教学:http://networkx.readthedocs.io/en/networkx-1.11/tutorial/
安装
pip install networkx
引入模块
import networkx as nx
print nx
无向图
例1:
#!-*- coding:utf8-*-
import networkx as nx
#linux系统下没有作图系统,需要如下生成图片
import matplotlib
matplotlib.use('Agg')
import matplotlib.pyplot as plt
#windows下直接
import matplotlib.pyplot as plt
G = nx.Graph() #建立一个空的无向图G G.add_node(1) #添加一个节点1
G.add_edge(2,3) #添加一条边2-3(隐含着添加了两个节点2、3)
G.add_edge(3,2) #对于无向图,边3-2与边2-3被认为是一条边
print "nodes:", G.nodes() #输出全部的节点: [1, 2, 3]
print "edges:", G.edges() #输出全部的边:[(2, 3)]
print "number of edges:", G.number_of_edges() #输出边的数量:1
nx.draw(G,with_labels=True) #nodes的标签加上
plt.savefig("wuxiangtu.png")
plt.show()
输出:
nodes: [1, 2, 3]
edges: [(2, 3)]
number of edges: 1
例2:
#-*- coding:utf8-*-
import networkx as nx
import matplotlib.pyplot as plt
G = nx.DiGraph()
G.add_node(1)
G.add_node(2) #加点
G.add_nodes_from([3,4,5,6]) #加点集合
G.add_cycle([1,2,3,4]) #加环
G.add_edge(1,3)
G.add_edges_from([(3,5),(3,6),(6,7)]) #加边集合
nx.draw(G)
plt.savefig("youxiangtu.png")
plt.show()
有向图
例1:
#!-*- coding:utf8-*-
import networkx as nx
import matplotlib.pyplot as plt
G = nx.DiGraph()
G.add_node(1)
G.add_node(2)
G.add_nodes_from([3,4,5,6])
G.add_cycle([1,2,3,4])
G.add_edge(1,3)
G.add_edges_from([(3,5),(3,6),(6,7)])
nx.draw(G)
plt.savefig("youxiangtu.png")
plt.show()
注:有向图和无向图可以互相转换,使用函数:
Graph.to_undirected()
Graph.to_directed()
例2,例子中把有向图转化为无向图:
#!-*- coding:utf8-*-
import networkx as nx
import matplotlib.pyplot as plt
G = nx.DiGraph()
G.add_node(1)
G.add_node(2)
G.add_nodes_from([3,4,5,6])
G.add_cycle([1,2,3,4])
G.add_edge(1,3)
G.add_edges_from([(3,5),(3,6),(6,7)])
G = G.to_undirected()
nx.draw(G)
plt.savefig("wuxiangtu.png")
plt.show()
注意区分以下2例
例3-1
#-*- coding:utf8-*-
import networkx as nx
import matplotlib.pyplot as plt
G = nx.DiGraph()
road_nodes = {'a': 1, 'b': 2, 'c': 3}
#road_nodes = {'a':{1:1}, 'b':{2:2}, 'c':{3:3}}
road_edges = [('a', 'b'), ('b', 'c')]
G.add_nodes_from(road_nodes.iteritems())
G.add_edges_from(road_edges)
nx.draw(G)
plt.savefig("youxiangtu.png")
plt.show()
例3-2
#-*- coding:utf8-*-
import networkx as nx
import matplotlib.pyplot as plt
G = nx.DiGraph()
#road_nodes = {'a': 1, 'b': 2, 'c': 3}
road_nodes = {'a':{1:1}, 'b':{2:2}, 'c':{3:3}}
road_edges = [('a', 'b'), ('b', 'c')]
G.add_nodes_from(road_nodes.iteritems())
G.add_edges_from(road_edges)
nx.draw(G)
plt.savefig("youxiangtu.png")
plt.show()
加权图
有向图和无向图都可以给边赋予权重,用到的方法是add_weighted_edges_from,它接受1个或多个三元组[u,v,w]作为参数,其中u是起点,v是终点,w是权重。
例1:
import networkx as nx
import matplotlib.pyplot as plt
G = nx.Graph() #建立一个空的无向图G
G.add_edge(2,3) #添加一条边2-3(隐含着添加了两个节点2、3)
G.add_weighted_edges_from([(3, 4, 3.5),(3, 5, 7.0)]) #对于无向图,边3-2与边2-3被认为是一条边
print G.get_edge_data(2, 3)
print G.get_edge_data(3, 4)
print G.get_edge_data(3, 5)
nx.draw(G)
plt.savefig("wuxiangtu.png")
plt.show()
输出
{}
{'weight': 3.5}
{'weight': 7.0}
经典图论算法计算
计算1:求无向图的任意两点间的最短路径
import networkx as nx
import matplotlib.pyplot as plt
#计算1:求无向图的任意两点间的最短路径
G = nx.Graph()
G.add_edges_from([(1,2),(1,3),(1,4),(1,5),(4,5),(4,6),(5,6)])
path = nx.all_pairs_shortest_path(G)
print path[1]
强连通、弱连通
- 强连通:有向图中任意两点v1、v2间存在v1到v2的路径(path)及v2到v1的路径。
- 弱联通:将有向图的所有的有向边替换为无向边,所得到的图称为原图的基图。如果一个有向图的基图是连通图,则有向图是弱连通图。
例1:弱连通
import networkx as nx
import matplotlib.pyplot as plt
#G = nx.path_graph(4, create_using=nx.Graph())
#0 1 2 3
G = nx.path_graph(4, create_using=nx.DiGraph()) #默认生成节点0 1 2 3,生成有向变0->1,1->2,2->3
G.add_path([7, 8, 3]) #生成有向边:7->8->3
for c in nx.weakly_connected_components(G):
print c
print [len(c) for c in sorted(nx.weakly_connected_components(G), key=len, reverse=True)]
nx.draw(G)
plt.savefig("youxiangtu.png")
plt.show()
执行结果
set([0, 1, 2, 3, 7, 8])
[6]
例2:强连通
import networkx as nx
import matplotlib.pyplot as plt
#G = nx.path_graph(4, create_using=nx.Graph())
#0 1 2 3
G = nx.path_graph(4, create_using=nx.DiGraph())
G.add_path([3, 8, 1])
#for c in nx.strongly_connected_components(G):
# print c
#
#print [len(c) for c in sorted(nx.strongly_connected_components(G), key=len, reverse=True)]
con = nx.strongly_connected_components(G)
print con
print type(con)
print list(con)
nx.draw(G)
plt.savefig("youxiangtu.png")
plt.show()
执行结果
<type 'generator'>
[set([8, 1, 2, 3]), set([0])]
子图
import networkx as nx
import matplotlib.pyplot as plt
G = nx.DiGraph()
G.add_path([5, 6, 7, 8])
sub_graph = G.subgraph([5, 6, 8])
#sub_graph = G.subgraph((5, 6, 8)) #ok 一样
nx.draw(sub_graph)
plt.savefig("youxiangtu.png")
plt.show()
二、其他函数
planted_partition_graph
planted_partition_graph(l, k, p_in, p_out, seed=None, directed=False)
函数详解:
Return the planted l-partition graph.
This model partitions a graph with n=l*k vertices in l groups with k vertices each. Vertices of the same group are linked with a probability p_in, and vertices of different groups are linked with probability p_out.
Parameters:
l (int) – Number of groups
k (int) – Number of vertices in each group
p_in (float) – probability of connecting vertices within a group
p_out (float) – probability of connected vertices between groups
seed (int,optional) – Seed for random number generator(default=None)
directed (bool,optional (default=False)) – If True return a directed graph
Returns:
G – planted l-partition graph
Return type:
NetworkX Graph or DiGraph
Raises:
NetworkXError: – If p_in,p_out are not in [0,1] or
具体例子
import networkx as nx
gene_net = nx.planted_partition_graph(50, 10, 0.2, 0.05, seed=42) # 50组,每10个,算是500个了吧
## 理解这是什么图
# ## 1.把图做出来
# nx.draw(gene_net)
# plt.savefig('test.png')
# plt.show()
# 2.查看nodes
# print gene_net.nodes()
#看到了0-499 的list,说明应该是 500个nodes,难道是 50 *10 ?
# 3.输出图的边值
# print gene_net.edges()
# 出来的是 0-499任意两个数的set。
# 其他属性
# print gene_net.degree()
# print gene_net.degree_histogram()
# print gene_net.density()
# print nx.info(gene_net)
# Type: Graph
# Number of nodes: 500
# Number of edges: 6566
# Average degree: 26.2640
# print nx.is_directed(gene_net)
# False
## 获得Nodes
# print len(nx.nodes(gene_net))
# 500
2.2 独立的node,即没有Node与其形成edge
import networkx as nx
G = nx.path_graph(4)
G.add_edge(5,6)
G.add_node(7)
G.add_node(8)
graphs = list(nx.isolates(G))
for one_part in graphs:
print(one_part)
结果:
7
8
2.3 连通子图,子图里面不需要任意两两之间相连
import networkx as nx
G = nx.path_graph(4)
G.add_edge(5,6)
G.add_edge(5,7)
graphs = list(nx.connected_component_subgraphs(G))
for one_part in graphs:
print(one_part.nodes)
结果:
[0, 1, 2, 3]
[5, 6, 7]
2.4 任意两点之间的联通
同时适用于有向图哦
G = nx.complete_graph(4)
for path in nx.all_simple_paths(G, source=0, target=3):
print(path)
[0, 1, 2, 3]
[0, 1, 3]
[0, 2, 1, 3]
[0, 2, 3]
[0, 3]
paths = nx.all_simple_paths(G, source=0, target=3, cutoff=2)
print(list(paths))
[[0, 1, 3], [0, 2, 3], [0, 3]]
说明:
source :起点Node
target: 终点node
cutoff: Depth to stop the search. Only paths of length <= cutoff are returned
2.5 最短path
G = nx.path_graph(5)
path = nx.all_pairs_shortest_path(G)
print(path[0][4])
[0, 1, 2, 3, 4]
2.6 最长path
不适用于环状的图
def longest_path(G):
dist = {} # stores [node, distance] pair
for node in nx.topological_sort(G):
# pairs of dist,node for all incoming edges
pairs = [(dist[v][0]+1,v) for v in G.pred[node]]
if pairs:
dist[node] = max(pairs)
else:
dist[node] = (0, node)
node,(length,_) = max(dist.items(), key=lambda x:x[1])
path = []
while length > 0:
path.append(node)
length,node = dist[node]
return list(reversed(path))
if __name__=='__main__':
G = nx.DiGraph()
G.add_path([1,2,3,4])
G.add_path([1,20,30,31,32,4])
nx.draw(G, with_labels=True,font_size=20)
# G.add_path([20,2,200,31])
print(longest_path(G))
三、案例:
import networkx as nx
from networkx.algorithms.approximation.clique import max_clique, clique_removal
G = nx.Graph()
G.add_edge(primer1, primer2)
nx.draw(G, with_labels=True)
plt.savefig('temp/network.png')
plt.show()
各种有可能的连通图的组合
cliques_primers1 = list(nx.find_cliques(G))
找到最大的连通图,然后去除图中的东西,接着找下一个图
cliques_primers2 = list(list(clique_removal(G))[1])
四、讨论:
1.加颜色
import networkx as nx
import numpy as np
import matplotlib.pyplot as plt
G = nx.Graph()
G.add_edges_from(
[('A', 'B'), ('A', 'C'), ('D', 'B'), ('E', 'C'), ('E', 'F'),
('B', 'H'), ('B', 'G'), ('B', 'F'), ('C', 'G')])
val_map = {'A': 1.0,
'D': 0.5714285714285714,
'H': 0.0}
values = [val_map.get(node, 0.25) for node in G.nodes()]
nx.draw(G, cmap=plt.get_cmap('jet'), node_color=values)
# nx.draw(G, cmap=plt.get_cmap('viridis'), node_color=values, with_labels=True, font_color='white') #其他颜色: PuBuGn , PuBuGn_r
plt.show()
参考资料
- http://stackoverflow.com/questions/13517614/draw-different-color-for-nodes-in-networkx-based-on-their-node-value
- http://www.cnblogs.com/kaituorensheng/p/5423131.html
- https://networkx.github.io/documentation/networkx-1.9.1/reference/generated/networkx.algorithms.components.connected.connected_component_subgraphs.html
- https://networkx.github.io/documentation/networkx-1.10/reference/generated/networkx.algorithms.isolate.isolates.html
- https://stackoverflow.com/questions/17985202/networkx-efficiently-find-absolute-longest-path-in-digraph
药企,独角兽,苏州。团队长期招人,感兴趣的都可以发邮件聊聊:tiehan@sina.cn
个人公众号,比较懒,很少更新,可以在上面提问题,如果回复不及时,可发邮件给我: tiehan@sina.cn
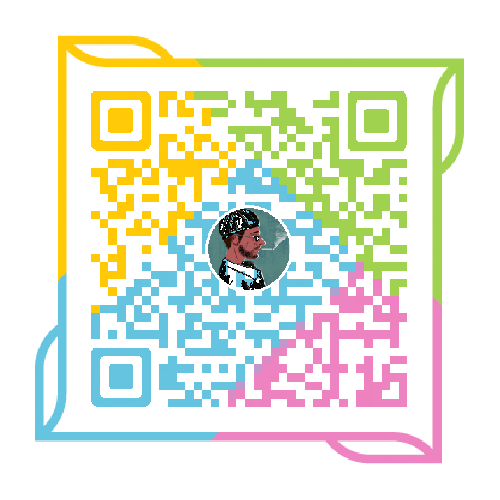